Use case – Ornikar tackles the insurance market
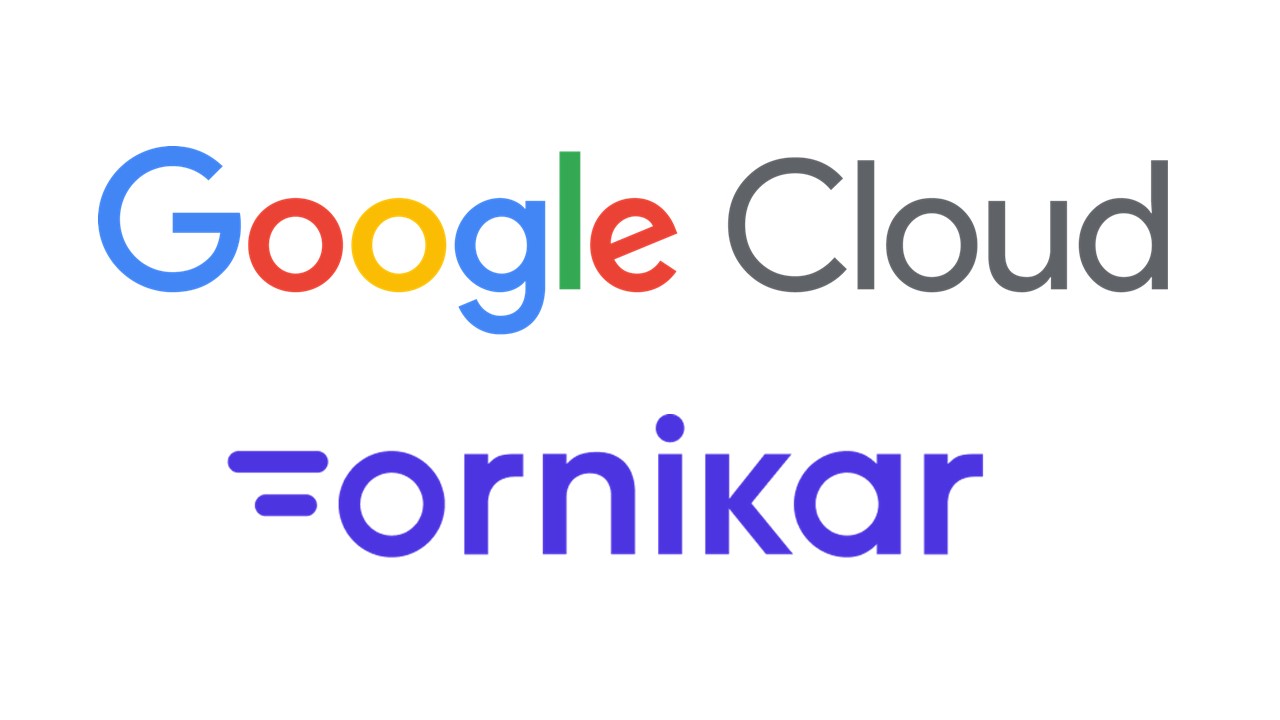
Ornikar is attacking the insurance market after having democratized the driving license market, capitalizing on its unprecedented knowledge of the 3 million young people who have passed through its driving school.
Ornikar intends to break the rents that still persist in the mobility sector, and this, in the service of young people and their emancipation, by creating the first car insurance that insures (really!) young people.
Ornikar is the reference platform for driving education in France which has revolutionized and democratized the sector, by removing the obstacles inherent in the customer experience: since its creation in 2013, the company has reinvented the car market -school by offering reduced prices (Ornikar has divided the price of the permit by 2!) and an experience facilitated by digital technology. It aims to establish itself as a leader in access to mobility and more broadly to the autonomy of young people by continuing its revolution with the creation of the first car insurance that capitalizes on driving training for young people. With more than 3 million students trained in its driving school, Ornikar indeed enjoys a major advantage.
If the first step of emancipation goes through the license, the second necessarily goes through auto insurance. Today, young drivers traditionally incur particularly high all-risk premiums, around 2000 euros per year, an exorbitant amount compared to their purchasing power. A price that insurers justify by the accidentology of novice drivers. In reality, this excessive pricing stems more from the lack of knowledge that traditional insurers have of the driving experience of young drivers.
How is this customer knowledge at the heart of the creation of a unique insurance model?
- The development of more suitable offers thanks to a better understanding of learning methods
Our digital learning path combined with a high volume of users provides us with unique knowledge of customer uses and behaviors. Starting with the possibility of developing for each of our students a model of their understanding of risks. Did he have a good perception of safety distances during his learning path? Does he tend to take for granted notions of security that are still insufficiently mastered? The axes that define knowledge of road risk are numerous.
For several years now, we have been using Machine Learning on this data, in order to enrich our pedagogy: for example, to indicate to a person whether or not they are ready to take the code review, based on their learning results. Our training exams being more demanding than the official exam, this indicator gives the student a more accurate assessment of his chances of success. Followed in near real time by the user, it allows him to approach the passage of the code more quickly and with confidence.
Our approach is based on the use of artificial intelligence, but always in collaboration with our educational experts. We thus establish indicators consistent with the state of the art available, in order to then assess their predictive potential.
The next axis of enrichment of our learning path involves the use of telematics in driving, capturing the movements of the vehicle in real time. These technologies allow us to improve the quality of feedback from the teacher to the student, with quantitative elements allowing him to support his advice.
- The development of proprietary accidentology models
Already a broker since 2021, we are now moving up the insurance value chain by integrating the entire customer relationship. This is how we launched in April 2023 a new offer on an MGA (Managing General Agent) insurer model, a model still not very common in France but which has proven itself in the UK.
Associating real accident risks with learning to drive, with data at such a level of completeness, is a first. This approach has great potential in terms of Data Science, and brings value to both of our offerings.
On the one hand, we can refine our education pathways, for example by suggesting specific modules to the students we identify as most at risk. We are thus strengthening our value proposition on the prevention of road risks, beyond the simple obtaining of a permit.
On the other hand, we can offer insurance more in line with the future behavior of our policyholders. We adapt our offers and our prices to their real situation, unknown to other insurers and which often leads them to excessive pricing.
We can indeed understand, thanks to the data provided by the tools for teaching driving, their approach to risk and road danger. Learning the rules of the road also plays a role, as does the attitude and reaction to failures. We can then correlate all of its elements of student understanding with the traditional criteria of the car market. Ultimately, this allows us to establish an average rate 20 to 25% below market rates.
This improvement in the understanding of young drivers is today an innovation and the sine qua non for transforming one of the densest and most dynamic retail markets in the insurance sector.
- The creation of new models at the service of the autonomy of young people
This new context of opportunities linked to customer knowledge encourages us more than ever to be data-driven in our development. In practice, the term data-driven is often limited to the meaning of “making decisions based on quantitative analyses”.
By recognizing the value of Data Science uses at the service of our users, we are questioning our past modes of operation. To prevent the formation of Tech and Data silos, it is now through co-design by the Back-End/Front-End and Data teams that we are drawing the future of our platform.
The Data architecture is also a crucial element for the ease of deployment of our Machine Learning systems. To do this, we benefit from the advice of the Google Cloud teams, all of our data being hosted on Google Cloud Platform. Having the right Storage Engines and the right Dataflows for putting our projects into production remains a key element in developing services with controlled infrastructure costs.
Finally, we regularly rethink the interactions between increasingly specialized roles – Machine Learning Engineers, Data Engineers, Analytics Engineers, Data Scientists, etc. – the involvement of all being essential to the success of our Data Science projects.
It is this operating structure that allows us to invent new models and relevant offers for the markets that we aim to disrupt!